From their use in smart TVs to smartwatches, the synthetic voices of AI assistants like Alexa and Siri have taken the world by storm. As of 2020, there were about 4.2 billion digital voice assistants in operation in devices across the globe. Forecasts for 2024 suggest that this number is projected to reach 8.4 billion— exceeding the world’s current population. And these voice assistants have come a long way.
No longer do voice assistants sound classically ‘robotic’. There seems to be an uncanny similarity between Google Assistant and all its regional accents and the accents of real-life native speakers. With the rate at which AI is advancing, it is soon expected that synthetic voices will be indistinguishable from human ones. This begs the question: What about synthetic voices makes them so human?
But before we delve into the nitty gritty of artificial speech, let’s clarify what synthetic voices are and what makes them ‘manufactured.’
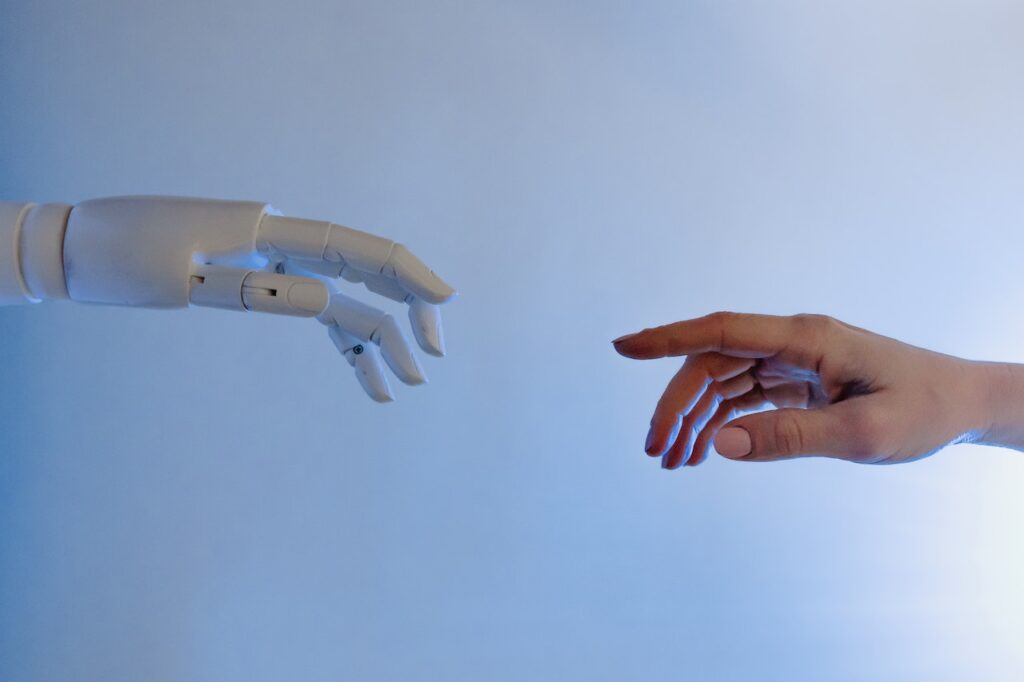
How synthetic voices are made
Synthetic speech is artificially (computer) produced human speech. What makes it synthetic? Can’t snippets of recordings of human speech just be edited to create ‘artificial’ speech? While that definitely counts as a form of synthesized speech, computers are far more elegant in how they go about generating artificial speech. Here are the two major ways they do so:
Concatenation synthesis
A considerable part of synthetic speech is created using something called ‘concatenation synthesis.’ It works as follows: phonemes — individual units of sound — of a speech recording are strung together and represented as waveforms. This form is ‘copied’ as a sonic waveform and the copy is processed to create an artificial render of human speech. The end result is raw audio data extracted from natural speech that mimics a human talking.
But concatenation synthesis has its shortcomings. The main drawback of this method is that its waveforms are segmented in a manner that limits capturing the precise details of natural speech. As short snippets of human speech are segmented into bites of audio, there is some room for tiny but only audible speech variations.
The result? That classically clunky and robotic sound you can probably imagine. So, the next phase of speech synthesis has seen the adoption of a new method to create synthetic speech, a technique so innovative that is largely responsible for the startling similarity of AI speech to human voices. These are called neural networks.
Neural Networks
Here’s the primary difference between neural networks and concatenation synthesis. The former creates waveforms as visual representations known as spectrograms, while its older variant creates audio waveforms. This difference matters because, unlike audio files, you get to capture intimate details of speech as a visual spectrum of frequency bands of a signal.
If the jargon is getting too much at this point think of a concatenation synthesis as a watercolor painting. It captures the essence of a scenic view (human speech) but not the crisp details. Think of neural networks as an oil painting with all the shadows, sharp lines, and rough edges working together to represent the same scene in a much more vivid way.
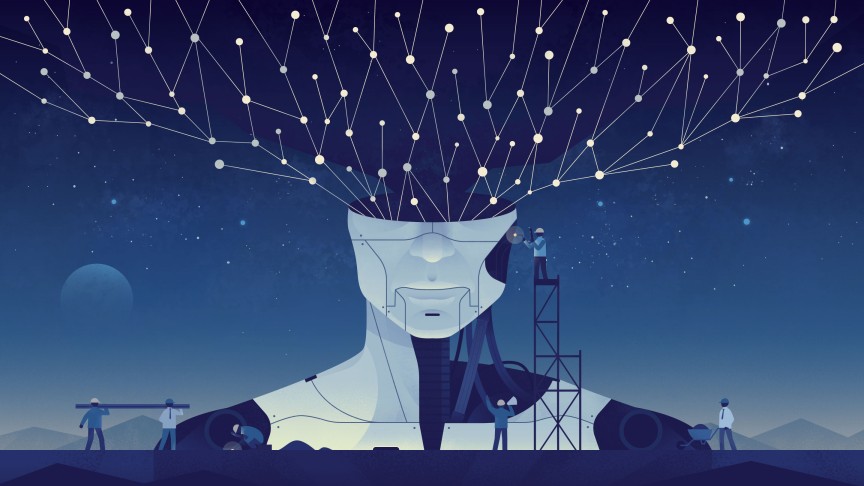
Hence, detail and texture are incredibly enhanced in the neural network method of artificial speech synthesis. But even something so advanced is not without its drawbacks. Both time and capital are required to neurally map speech. A study by the BBC, at least 24 hours of a single voice recording costing well over £500 needed to be poured into a program to create a natural map for that one voice.
Imagine trying to scale this up for more voices, accents, and languages. In other words, how can we capture the textures, intonations, and accent differences for speech efficiently?
Where synthetic voices are going
It’s clear that neural networks, even with their drawbacks, have been a game changer for synthetic speech. But this has only been the beginning. Today, the innovations surrounding synthetic voices look like this:
Fast voice cloning
Hop on the internet and you can find online packages that exist solely for real-time voice cloning using minimal sections of audio. You can ‘clone’ an entire voice with a recording of just a few seconds, making this process near instantaneous in comparison to neural networks. But everything that sounds too good to be true usually is. Fast voice cloning, which offers speed, fall short when it comes to utility, particularly because of its inability to retain accents. The end result is , therefore, not very good for production.
Visually, this method can be compared to taking a photo of scenery: fast, cheap, instant, and copyable. But it is a pixellated version of the real thing so it might lack depth and versatility. And yet, even fast voice cloning shows off one of the new technologies governing synthetic voices: The fact that you do not need to start from scratch to create new voices. Once you have a working cloned voice, that can serve as the base to create more voices in different timbres. By extracting the ‘fingerprint’ of a voice through a process called embedding, you can simply tweak this artificial version — similar to photoshopping an image — to get one or many unique variants.
Deep convolutional neural networks:
Deep convolutional neural networks are neural networks with the necessary complexity needed to capture the essential accent-related details. As a visual example, think of this technology as a hyper-realistic oil painting. As there aren’t any recurrent units in this network, it can be trained quicker and less expensively than standard neural networks.
Although state-of-the-art text-to-speech technology can still perform a better job than fine-tuned neural networks, the latter is quicker, cheaper, and requires just a couple of hours of voice recording to generate a sonic model.
Where Dubverse fits in
A good case study of using the latest and best in synthetic voice technology is Dubverse.
Dubverse’s proprietary AI models generate synthetic voices from scratch using just one hour of data, staggeringly low investment in comparison to the standard of 30 hours of data. But that’s not all. The model is able to build and sustain the texture details of the voice in multiple different languages. All this in just two days of receiving data.
Here is some of what Dubverse is aiming to solve such that its synthetic speech technology can get even sharper
- Emotion and style transfer: How can we capture the emotional components of human speech as well as the unique styles attributed to individual speakers?
- Cross-lingual voice cloning: Can we use a single language source (say English only) to clone artificial voices across multiple different languages? This feature is now available.
- Real-time speech synthesis: Is it possible to synthesize artificial speech in real-time for live content?
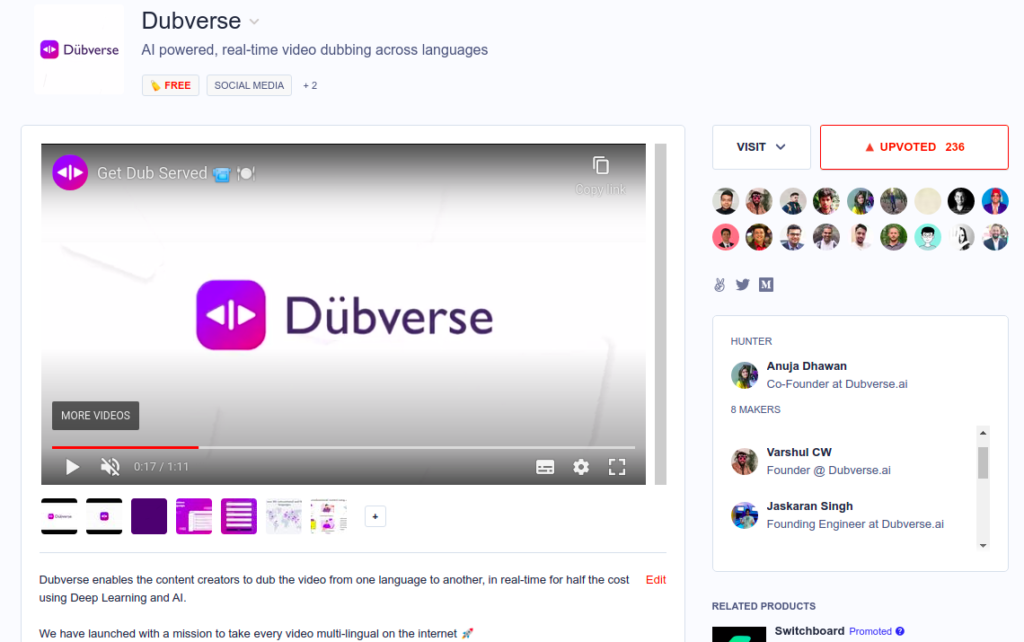
We recently launched our web app on Product hunt.
Read more about Dubverse’s journey from day 1.
Final thoughts: The need for synthetic dubbing
Being able to create a human-like speech that is natural-sounding from the ground up has been the goal of speech enthusiasts and scientists for decades on end. In the media world, synthetic speech offers incredible convenience, especially for brands who have their hands full and need to market their content in different languages to grow their audience.
In the non-media world, this technology serves the noble purpose of aiding a major segment of the population. Differently abled individuals with visual impairments or with an inability to verbally communicate can greatly improve their quality of life from the easily accessible text-to-speech technology of synthetic speech. With every passing year, the technology becomes more publicly available, paving the way for multilingual content to cast its net across a broader audience.